Building The Experimentation Engine In Your Organization – The New Leader’s 30-60-90 Day Plan
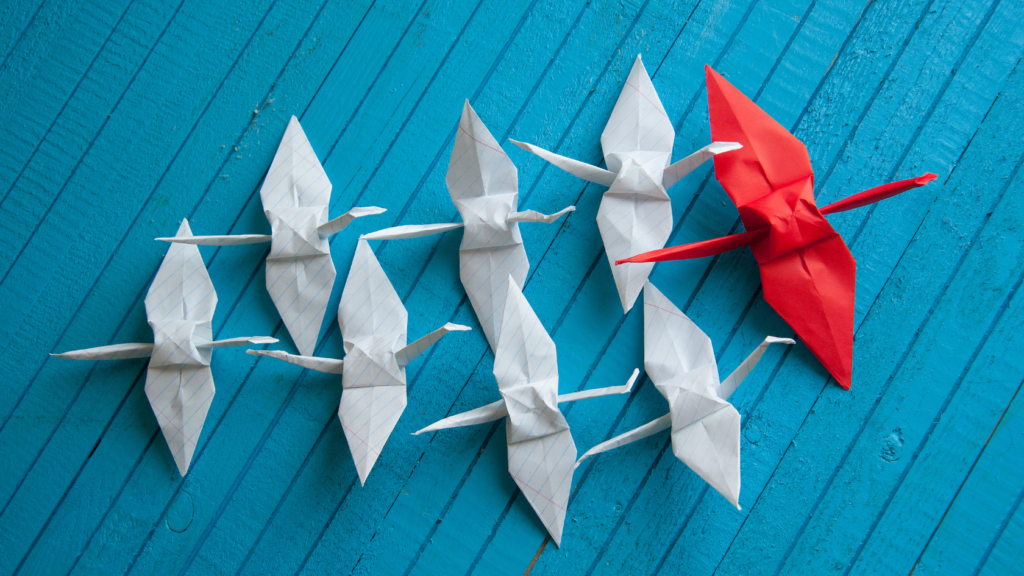
Congratulations! You have just been hired (or promoted) as an Experimentation Leader / Manager / Lead and are raring to go! The real work starts now and getting to value is all about speed and doing things correctly.
But where do you start? What should you tackle first?
As an Experimentation Lead, your role might involve bringing order to the chaos of ad hoc testing and building a standardized, scalable, and trustworthy experimentation framework across the business. If you’ve recently taken on a similar role or are looking to improve your company’s experimentation practices, you’ll need a clear roadmap to guide your efforts.
You won’t need convincing about the fact that companies that embrace experimentation can quickly validate ideas, optimize products, and respond to market changes with confidence. But what happens when experimentation practices across an organization are inconsistent, unreliable, or poorly understood? This is a challenge many large enterprises face, and it’s a problem we (at Effective Experiments) have seen first hand at many companies.
In this post, I’ll share a 30-60-90 day plan that you can follow to transform experimentation within your organization. Whether you’re starting from scratch or looking to improve and fix existing processes, this plan will help you build a self sustaining experimentation engine within your organization where data and experimentation drive decision-making, and every test is designed to generate actionable insights.
Let’s dive into the roadmap!
First 30 Days: Understand and Assess
Objective: Gain a comprehensive understanding of the current experimentation landscape and build relationships with people in the business.
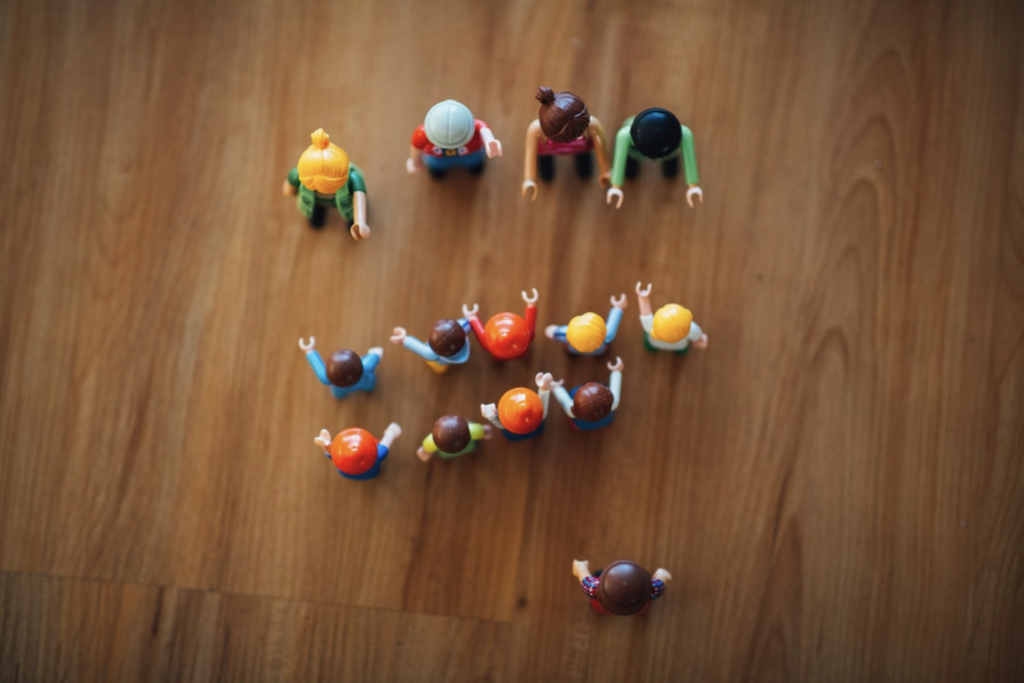
1. Meet with Key Stakeholders:
Your experimentation efforts will be of service (or disservice) to a lot of different stakeholders in the business. Your job is to get to know them better not just on a transactional level but on a personal level.
Who to meet: Schedule meetings with leaders from product, marketing, data science, engineering, and any other departments that rely on experimentation. Prioritize those teams that are running the most critical or frequent experiments but also pay close attention to those that have never run experiments or are against the idea of it.
What to discuss:
In this conversation, it is critical to gauge the understanding of the person you are speaking with when it comes to experimentation, the processes, the outcomes and use of insights.
Ask about their current experimentation efforts, pain points, and what they expect from a more unified experimentation approach. Or alternatively, why they wouldn’t want to embed an experimentation approach.
Use this time to find out what success looks like to them (e.g., faster decisions, more trustworthy results, higher impact experiments).
You can discuss how experimentation fits into their overall strategy and decision-making processes.
Want a handy way to track every person you speak with so you can track their understanding and pain points?
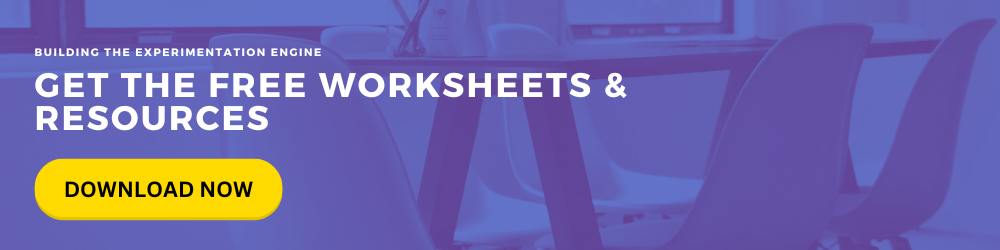
2. Audit Current Experimentation Practices:
Before creating a plan on how to improve the experimentation practice, you have to know the current situation. What does experimentation look like in the organization? What is the maturity of the individuals involved? All of these facts will help shape a strategy to improve it.
How to approach it:
Start by conducting a comprehensive review of the experimentation practices across teams. This includes reviewing past and current experiments, analyzing documentation, and assessing the rigor of each experiment. Make note of how robust or weak the practices are.
Go into every minute detail. Gather data on how experiments are designed, what metrics are used to define success, and how results are communicated and acted upon.
Get deeper with the statistics and assess whether proper statistical techniques (e.g., sample size calculation, statistical significance) are being applied consistently. There’s no shame here but you may want to bring in someone external if you lack the knowledge and statistics.
Identify any common pitfalls, such as poorly defined hypotheses, improper sample sizes, or failure to account for confounding factors.
A common challenge that most new Experimentation leaders run into is that all this information is scattered all over the place or there are poor data management practices which make this part of the job harder
Tools to use: You might need to request access to experiment logs and documentation (whether that’s in spreadsheets or a dedicated platform like Effective Experiments) analytics dashboards, or A/B testing platforms that different teams are using. You will need to break it down for each individual and team to gauge their maturity.
Outcome: Create a report highlighting the state of experimentation practices, noting areas of strength and opportunities for improvement.
Want an easy to use report template to audit the experimentation ?
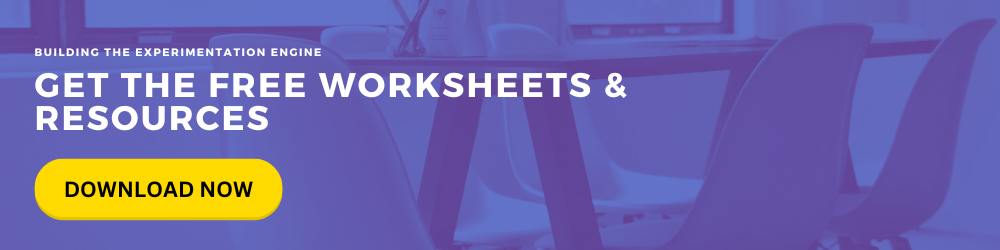
3. Map Out Experimentation Landscape:
No two experiments are the same and this is down to the variance in capabilities and maturity of the people involved. Never take experiments at their face value. Dig deeper to find out the gaps and opportunities.
How to approach it:
You will want to create a visual map or document that identifies all teams and individuals conducting experiments, along with a description of the types of experiments they’re running (e.g., A/B testing, multivariate testing, causal inference studies, quasi tests).
Be sure to include the tools each team is using for testing (e.g., in-house tools, third-party platforms like Optimizely or Google Optimize). Capture details on key variables, such as experiment cadence, how results are documented, and whether they follow a standardized process.
Outcome: This map will serve as a comprehensive view of the organization’s experimentation landscape, helping you identify where consolidation or streamlining may be necessary. It will also show you the gaps and highlight any teams that are not yet experimenting but could benefit from doing so.
4. Evaluate Current Tooling and Infrastructure:
How to approach it:
Perform a deep dive into the existing experimentation tools and platforms used across the company. These might include A/B testing platforms, feature flagging tools, and analytics platforms.
Assess whether these tools support the company’s needs in terms of scale (i.e., how many tests can run concurrently), ease of use (i.e., can non-technical teams use them?), and robustness (i.e., do they allow for proper statistical analysis?).
Consider if the tools allow for cross-team collaboration, proper version control, and seamless as well as reliable reporting. Examine whether the current infrastructure can handle more advanced testing methods, such as multivariate tests or personalization experiments.
Outcome: Generate a list of strengths and weaknesses for the current experimentation stack. Identify whether there is a need for additional tools or upgrades to support more scalable and reliable experimentation.
5. Build Relationships and Gather Feedback:
How to approach it:
Beyond formal meetings, invest time in informal interactions with team members to better understand the company culture and how experimentation is viewed across the business. Establish yourself as a trusted resource by offering quick, actionable insights based on your initial findings.
Actively listen to team members’ concerns about past experimentation failures or successes and gather feedback on what they would like to see improved.
You will also need to find support and backing from senior management without whom, any exercise in change will prove to be fruitless. They will help set remits and requirements on teams and individuals who you may not have direct authority over. Change is tough and often teams may reject your recommendations simply because you don’t have any authority over them.
Outcome: Building strong relationships early on will set the foundation for gaining buy-in when you start to introduce standardized processes and changes. These relationships will also help you identify potential experimentation champions within each team.
6. Identify Quick Wins:
How to approach it:
Look for low-effort, high-impact opportunities to make immediate improvements. For example, if documentation practices are inconsistent, create a simple, standardized template that all teams can use.
Offer to review ongoing experiments to identify quick adjustments that could improve quality, such as ensuring proper randomization or clarifying success metrics.
If multiple teams are using different tools, look to centralize tools in one area to test for improved efficiency as well as better oversight and the ability to standardise the processes.
Consider hosting a short training or knowledge-sharing session on best practices for experimentation, tailored to address some of the most common issues you identified during the audit.
Outcome: By delivering quick, tangible improvements early on, you will build credibility and demonstrate the value of your role. These quick wins will also create momentum for more substantial changes down the road.
Word of caution: Quick wins should never be about encouraging speed by sacrificing quality. Quite often it may seem easier to get people to run tests no matter the quality but it can backfire in the long term.
Next 30-60 Days: Plan and Design
Objective: Develop a standardized experimentation framework, pilot it with select teams, and lay the groundwork for scaling across the organization.
1. Define Experimentation Best Practices:
Establish core principles:
Based on your findings from the first 30 days, define a clear set of best practices that will standardize experimentation across the company.
These practices should address key aspects of experimentation, such as hypothesis development, processes, proper randomization, statistical rigor, and the use of control groups.
Create clear definitions and use cases documenting how teams should decide between and select different experiment types (e.g., A/B testing, multivariate testing, cohort analysis) depending on the context.
Standardize success metrics:
Create a set of guidelines for defining success metrics. Ensure that all teams use metrics that are aligned with business objectives and can be consistently tracked across experiments.
Encourage the use of primary metrics (the main goal of the experiment) and secondary metrics (which provide additional context or insight into user behavior) as well as guardrail metrics.
Ensure teams avoid common pitfalls like over-relying on vanity metrics (e.g., pageviews) that don’t provide meaningful insight into product or business performance.
Create a documentation framework:
Develop a standardized template for teams to document their experiments, including sections for hypothesis, experiment design, sample size, results, and learnings.
Ensure that this documentation is comprehensive but simple enough to encourage use by all teams. This is where you will have to strike a balance. You must have documentation that not only captures the technical aspects of the test and the outcomes but also information that relates to the business.
A good exercise to envision what you should document is this – If you were to review and search for insights within your experiment repository, what are all the insights would you look for? Now – are they findable? Are they tracked or are you spotting gaps.
Create a centralized repository where all experimentation documentation is stored for future reference, helping teams avoid reinventing the wheel or running redundant tests.
Effective Experiments helps you manage all your experimentation activities in one single platform and comes out of the box with industry standard workflows and process built in.
Try out our self serve demo.
Outcome: A clear, standardized experimentation framework that can be used consistently across all teams to improve the quality and trustworthiness of experiments.
2. Design a Centralized Experimentation Process:
Create a centralized process for planning, executing, and analyzing experiments which includes guidelines for experiment design, hypothesis generation, sample size calculation, and statistical analysis.
Make it a standard practice to establish a review and approval process for experiments.
3. Pilot the New Experimentation Process:
Don’t roll this change out without any strategy. Select a few teams to pilot the standardized experimentation process that are more amenable and willing to work with you or ones who have been given the remit by senior management.
Work closely with these teams to refine the process based on feedback. Collect and analyze the results of these pilot experiments to demonstrate the value of the new approach.
4. Develop Training and Resources:
Create training materials, workshops, and documentation to help teams adopt the new experimentation practices. Provide resources like experiment design templates, statistical tools, and guidelines for interpreting results.
5. Enhance Tooling and Infrastructure:
If needed, begin the process of evaluating and selecting new experimentation tools or platforms. Work with IT and data teams to ensure that the experimentation infrastructure is robust and scalable.
6. Establish a Knowledge-Sharing Community:
Create a community of practice for experimentation where teams can share learnings, challenges, and successes.
Set up regular meetings or a forum for discussing experiments and exchanging ideas.
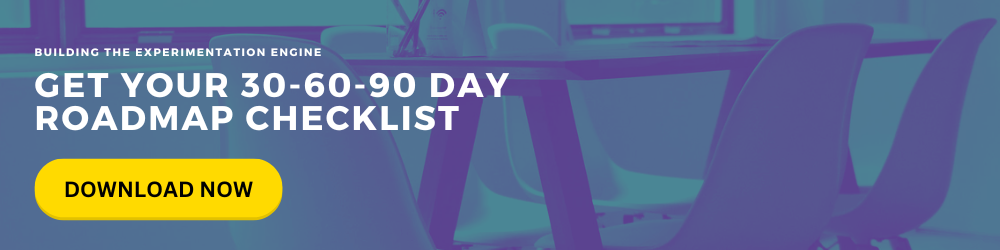
Next 60-90 Days: Implement and Scale
Now the rubber hits the road and you can scale what has worked and discard what hasn’t. Scaling across multiple teams can be challenging and it is important to remember that you will have to get senior leadership backing.
Objective: Roll out the standardized experimentation framework across different teams in the organization and measure its impact.
1. Roll Out Standardized Practices:
Expand beyond pilot teams:
Now the time has come to start rolling out the standardized experimentation framework across all teams in the organization, building on the success of the pilot program. Prioritize and pick the teams based on the volume or importance of their experiments, ensuring those with the highest impact are supported first.
Create tailored onboarding:
You may have to develop a structured onboarding process for teams that weren’t part of the pilot. Offer tailored training sessions and workshops to ensure these teams are fully equipped to adopt the new processes. Provide them with a clear, step-by-step guide for each phase of experimentation—hypothesis generation, design, execution, analysis, and reporting.
Offer ongoing support:
Ensure you or your team is available for ongoing support, especially for teams that are new to rigorous experimentation. Set up office hours or designate “experiment mentors” who can answer questions and review experiment designs.
Outcome: The majority of teams adopt the new standardized experimentation practices, leading to more consistent, high-quality experiments across the organization.
2. Monitor Adoption and Impact:
There’s no point putting in all the effort onboarding and training if you don’t keep a close eye on what follows. Are people and teams actually onboarding and more importantly improving in their practice?
Track adoption metrics:
Develop a set of metrics to track how well the new framework is being adopted. This could include:
- Number of teams using the new process.
- Number of experiments being conducted per team.
- Quality of experiments being conducted by the team
- Quality of Hypothesis
- Time saved in designing and analyzing experiments.
- Improvement in the quality of insights generated from experiments (e.g., more actionable results).
Additional Reading:
Experimentation Program Metrics Every Manager Must Track
Tracking Experiment Quality Using A Health Scorecard
Collect feedback from teams:
Actively seek feedback from teams to understand their experiences with the new process. Set up regular check-ins or surveys to capture both qualitative and quantitative feedback. It is important you quickly identify any bottlenecks or pain points that are slowing down adoption or leading to inconsistencies in execution.
Focus on understanding whether the framework is helping teams achieve their goals, such as faster decision-making, better validation of ideas, or higher business impact from experiments.
Outcome: You’ll have a clear understanding of how well the framework is being adopted and whether it is delivering the expected benefits, allowing you to make informed adjustments.
3. Refine and Optimize:
KeepIterating based on feedback:
Use the feedback and data you’ve collected to make iterative improvements to the framework. This might involve:
- Adjusting the documentation process to make it more user-friendly. (You may also have to resist changing this too much)
- Simplifying statistical guidelines if teams are struggling with complexity.
- Tweaking the approval process to reduce bottlenecks.
- Ensure that the framework is flexible enough to accommodate the evolving needs of different teams.
Address resistance:
If certain teams are resistant to change or struggling to adopt the new framework, work with them to understand their concerns. Offer additional training or support where needed.
Emphasize the benefits of a standardized process, such as improved decision-making, faster experimentation cycles, and more trustworthy results.
Outcome: The experimentation framework evolves based on real-world usage, becoming more efficient, user-friendly, and aligned with the needs of teams. Resistance is addressed, and adoption rates increase.
4. Expand Experimentation Capabilities:
Introduce advanced techniques:
Now that teams are familiar with basic A/B testing and multivariate experiments, introduce more advanced experimentation techniques. This could include:
- Personalization experiments: Tailoring experiments based on user segments.
- Sequential testing: Allowing teams to analyze results over time and stop tests early if necessary.
- Machine learning-driven experiments: Using AI to optimize test designs and personalization at scale.
Cross-team experimentation:
Encourage cross-team collaboration to run experiments that span multiple functions (e.g., a product team running an experiment in collaboration with marketing). Develop a process for sharing resources and learnings across teams to ensure that experiments are aligned and don’t overlap unnecessarily.
Expand to other business areas:
Explore opportunities for experimentation beyond the most common areas (product and marketing). Encourage teams in customer service, sales, or even HR to experiment with process improvements or new initiatives.
Outcome: Experimentation becomes more sophisticated, expanding into new areas and delivering even more value to the business.
5. Build an ecosystem for Experimentation to thrive:
Embed experimentation in decision-making:
Work with leadership to ensure experimentation is fully embedded into the decision-making process. Encourage teams to validate ideas and assumptions through experiments before making strategic decisions.
Promote a mindset of learning through failure. Ensure that teams feel comfortable running experiments even if they result in negative or unexpected outcomes, as these are valuable learning opportunities.
Recognize and celebrate success:
Recognize teams that have successfully adopted experimentation practices and delivered impactful results. This could involve public recognition during company meetings, in newsletters, or even internal awards for “Best Experiment” or “Most Innovative Use of Experimentation.”
Celebrate both successful and failed experiments that led to valuable insights, reinforcing the idea that all tests contribute to business learning.
Leadership engagement:
Work with senior leadership to ensure ongoing support for experimentation as a core part of the company’s strategy. Encourage them to ask for data and insights from experiments when reviewing major initiatives or strategies.
Outcome: A strong culture of experimentation takes root across the organization, where teams are encouraged to test, learn, and iterate as part of their day-to-day operations.
6. Measure Success and Report:
Track key success metrics:
Define and track key metrics that measure the success of the new experimentation framework, such as:
- Time to decision-making (reduction in time due to faster, data-driven insights).
- Experimentation velocity (increase in the number of experiments being run across the company).
- Business impact (e.g., revenue uplift, customer engagement improvements, or cost savings resulting from experiments).
- Regularly review these metrics with key stakeholders to assess how well the new processes are contributing to the overall business goals.
Regular reporting:
Create a standardized reporting cadence for sharing experimentation insights with leadership and teams. This could include monthly or quarterly reports summarizing experiment outcomes, business impact, and process improvements.
Highlight success stories whilst also emphasising key insights and learnings from various teams to keep the momentum going.
Outcome: You’ll be able to demonstrate the tangible value of experimentation to the business, helping to secure ongoing support and resources for further experimentation initiatives.
By the end of the 60-90 day phase, you will have successfully implemented the standardized experimentation framework across the organization, tracked adoption, refined processes based on feedback, and fostered a company-wide culture of experimentation. Additionally, you’ll have positioned experimentation as a key driver of business strategy and decision-making.
This phase is critical in ensuring that the changes you’ve implemented are sustainable and that the organization continues to evolve and optimize its experimentation capabilities.
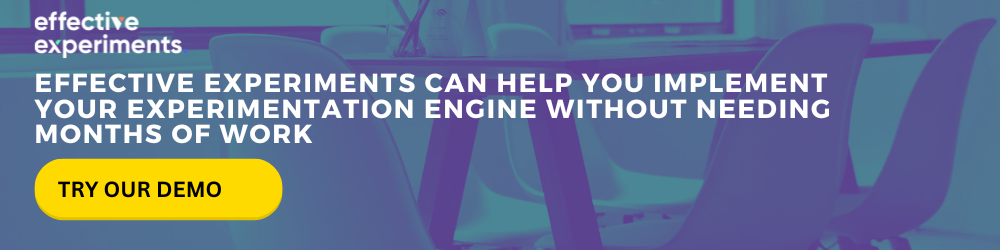