Vanity metrics in Experimentation Programs Pt.1
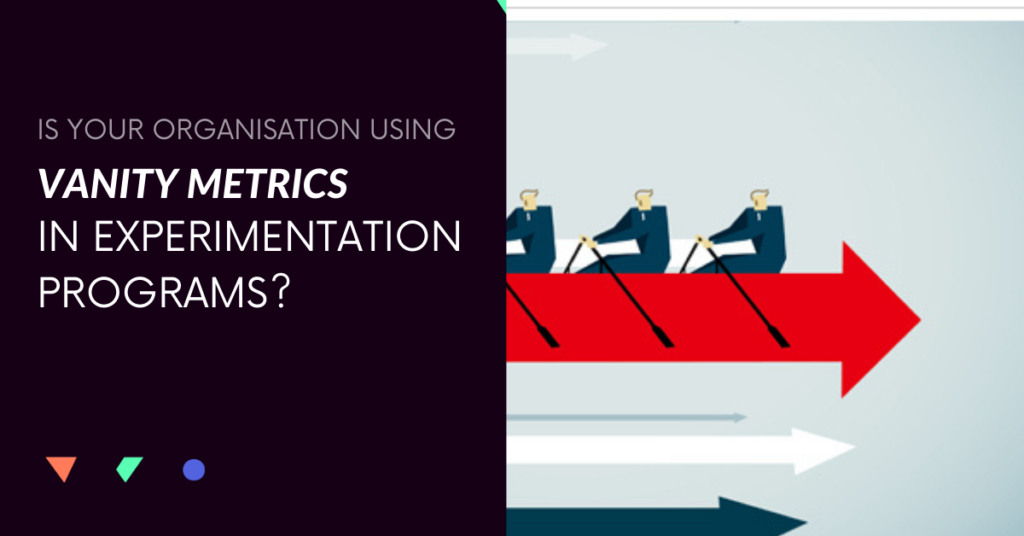
In this series of three articles, we’re going to question some of the “well intentioned” practices in the industry in setting certain KPIs and metrics to determine the success of a program. We will show you why they don’t work in practice and often hinder the real growth of an experimentation program. We will also give you a better alternative. Ultimately, we are aiming to change the mindset of those in senior management who are the ones that set such goals and improve the way experimentation programs are managed
Experimentation teams are stuck on a hamster wheel as a result of decisions made in the setup and rollout of testing across the organisation. Growing a culture of experimentation is the new “in thing” for a lot of organisations as they believe (rightly so) that without it, their quest to create an experimentation first mindset will run out of steam.
When reviewing the setup of a company’s experimentation setup, process and roll out, we have come across the metrics and KPIs that are used as a barometer of the programs ongoing success.
But, unfortunately these vanity metrics are used by companies without realising that by using them as goals and targets they are sending their experimentation programs down the wrong path.
The most commonly used metric is
“The number of experiments per month/quarter/year”
In principle, Number of experiments in a certain time period compared to the previous time period should tell us if a program is on the right track and growing at the right pace. After all, if the number of experiments this time period is higher, it indicates that more work was done, more insights gained and also points to other factors improving. This could be more resources and personnel running experiments. It could be that there’s more budget and an increased appetite for testing.
One would even argue that it’s a good starting point for a team to use a target when they first get going. Or else, how would they know what to aim for.
All of this looks good on paper until you start looking a bit deeper.
Compare two time periods.
50 experiments in Quarter 1 vs 100 experiments in Quarter 3.
Good news. We doubled the number of experiments and the milestone was hit.
However, it doesn’t tell you a lot more than that.
Some of the common areas that we look into during our organisation deep dive in the Experimentation Ops Audit are –
- Did the experiment have a valid hypothesis?
- Was this a random experiment or did it have a solid foundation in research?
- Did the experiment pass QA?
- Did the experiment have a solid test design?
- Were the results reported accurately and without bias?
- Were the insights gathered as a result of that experiment useful to the business?
- What business impact can be attributed to the experiment?
Marketing KPIs and data is susceptible to being manipulated, segmented or sliced and diced to tell whatever story the marketer wants you to hear.
Put a KPI in front of them and they are likely to find ways to game it just to hit that KPI. It happens frequently. It may or may not happen with malice but it will certainly happen especially if they’re close but not close enough to hitting that target.
No two experiments are alike. They can vary in complexity, time to production, potential issues with legal/branding etc and a variety of other factors that could impact it.
If the only metric is the number of metrics, what’s stopping the CRO from running experiments that don’t align with business goals or experiments that are basic and didn’t really have much thought put into it.
Redesigning “Number of Experiments” as a better metric
This one is especially written for the attention of CMOs and VPs of Marketing, not necessarily for the CROs or Experimentation Leads.
Why? Because if an organisation cares about Experimentation beyond the CRO silo, the VPs and CMOs or other C-level have to be actively involved. That begins with setting the right metrics. You also want someone who is detached from the outcome to be in charge of it.
Number of experiments can be useful when it comes with what we call Guardrail metrics.
Guardrail metrics ensure that you set standards and guidelines on how to evaluate each KPI predictably.
An experiment can have many quality indicators and creating an experiment scorecard allows you to determine whether the test is good, needs work or plain bad.
Each experiment scorecard needs to be set independently, vetted and reviewed. This removes bias or conflict of interest when managing the KPI.
This is what organisations should look at setting as a KPI
“Number of experiments run that meet or exceed a quality score of ____”
This encourages the CROs to focus on correct process and keeping an eye on the quality of the experiment and not just ticking a box to say they have completed 1 experiment.
Focus on the quality will embed better behaviours and root out bad experimentation reporting practices.
In part 2, we will look at another vanity metric that’s been used quite often.